AI’s Deep Research Boom: Unlocking Science’s Secrets and Your Role in It
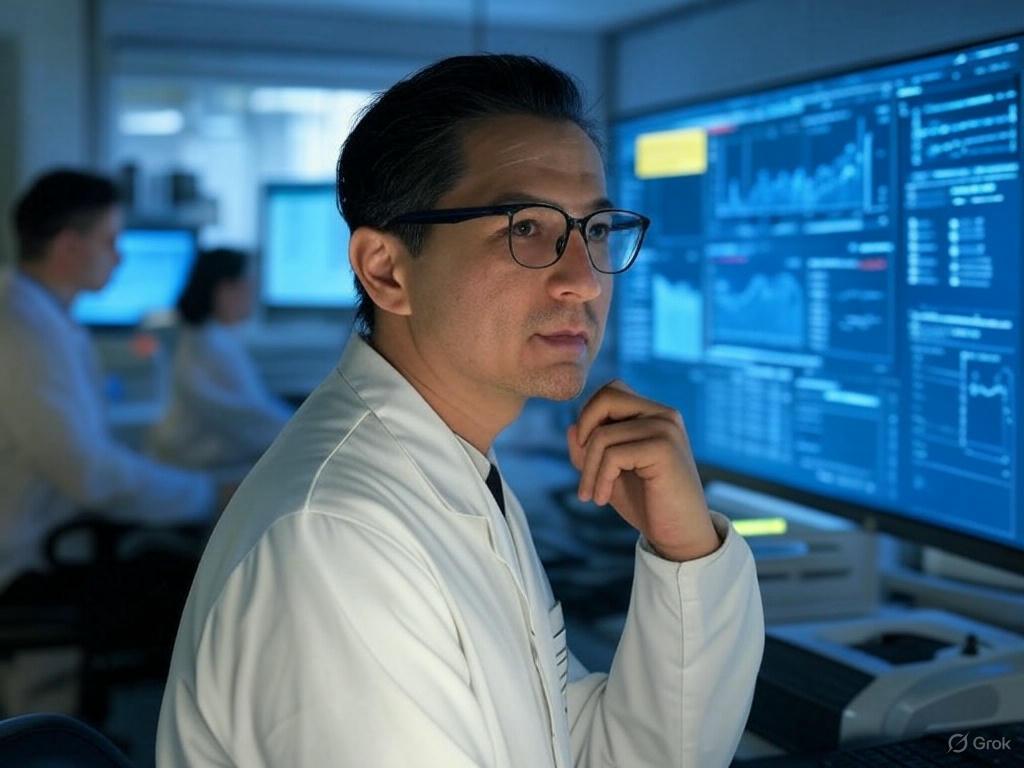
Key Points
- Research suggests AI is driving a "deep research trend" by enhancing scientific discovery, particularly in drug development, climate modeling, and genomics.
- It seems likely that AI accelerates research by analyzing vast data, identifying patterns, and predicting outcomes, making science faster and more cost-effective.
- The evidence leans toward AI enabling non-experts to contribute via citizen science projects like iNaturalist and Zooniverse, democratizing research.
- There’s growing interest in AI’s role in solving global challenges, but ethical and regulatory debates continue around data privacy and AI authorship in science.
What is the Deep Research Trend in AI?
The "deep research trend" in AI refers to how artificial intelligence is transforming scientific research by enabling deeper, faster, and more comprehensive analysis. AI acts like a super-powered assistant, processing massive amounts of data to identify patterns, make predictions, and even design experiments that were previously too complex or time-consuming for humans. This trend is particularly evident in fields like drug discovery, climate science, and genomics, where AI helps tackle some of the world’s biggest challenges.
For example, AI can simulate how molecules interact to find new drugs, model climate patterns to predict weather events, or analyze genetic data to understand diseases. This isn’t just about speed; it’s about uncovering insights that might be missed by human researchers, making research "deeper" in scope and impact.
Why Was This Trend Necessary?
Traditional scientific research can be slow and expensive, often limited by the ability to process and analyze large datasets. AI addresses these limitations by accelerating discovery, reducing costs, and opening new research avenues. For instance, developing a new drug can take years and billions of dollars, but AI can shorten this timeline by predicting which compounds are most likely to work. Similarly, climate scientists use AI to model complex systems, helping us better understand and combat climate change.
This trend is necessary because it helps address urgent global issues, like health crises and environmental threats, at a pace that matches their urgency. It also makes research more accessible, allowing non-experts to contribute through citizen science projects, democratizing science.
How Can You Start Using AI in Research?
Even if you’re not a scientist, you can get involved through citizen science projects that use AI. Platforms like iNaturalist (iNaturalist) let you upload photos of plants and animals to help train AI models for species identification. Zooniverse (Zooniverse) offers projects where you can classify data, like identifying galaxies or transcribing historical documents, aiding research efforts.
For those curious to learn more, online courses or popular science books can provide insights into AI’s role in science. If you’re a business owner or hobbyist, explore how AI tools can enhance your work, such as using AI for data analysis in marketing or personal projects.
Survey Note: Exploring the Deep Research Trend in AI and Its Implications
This survey note delves into the concept of the "deep research trend" in AI, focusing on its current state, significance, and practical applications, particularly for non-technical users. It aims to provide a comprehensive overview, ensuring readers are informed, excited, and ready to engage with this transformative technology. The content is tailored for amateur AI users, breaking down complex concepts into accessible language and offering actionable steps for participation.
Understanding the Deep Research Trend in AI
The term "deep research trend" in AI likely refers to the growing use of artificial intelligence to enhance scientific research, enabling deeper and more comprehensive analysis than traditional methods. This trend is driven by AI’s ability to process vast datasets, identify patterns, and make predictions, revolutionizing fields like drug discovery, climate science, and genomics.
AI in scientific research acts as a super-powered assistant, capable of tasks that would take humans years to complete. For instance, it can simulate molecular interactions to find new drugs, model climate patterns for better weather predictions, or analyze genetic sequences to uncover disease correlations. This "deep" aspect comes from AI’s capacity to uncover insights that might be missed by human researchers, making research more thorough and impactful.
This interpretation aligns with current discussions in AI, where the focus is on using advanced technologies to tackle complex, data-intensive problems. For example, a Microsoft article from December 2025 highlights AI’s role in scientific research as one of six key trends, noting its potential to address pressing global concerns like climate change and healthcare (Microsoft News Center 6 AI trends you’ll see more of in 2025).
Why This Trend Emerged and Its Necessity
The deep research trend in AI emerged due to the limitations of traditional research methods, which can be slow, costly, and constrained by human capacity to analyze data. Scientific research often involves processing massive datasets, such as genomic sequences or climate data, which can overwhelm human researchers. AI addresses this by accelerating analysis, reducing costs, and enabling new research avenues.
For example, developing a new drug traditionally takes over a decade and billions of dollars, but AI can predict which compounds are most likely to succeed, potentially halving the timeline. Similarly, climate scientists use AI to model complex systems, providing insights into deforestation, ice melt, and weather patterns, crucial for combating climate change (Fast Data Science AI in science and research).
This trend is necessary because it aligns with the urgency of global challenges. Health crises, environmental threats, and technological advancements demand faster, more efficient research. AI not only speeds up discovery but also democratizes science by allowing non-experts to contribute through citizen science projects, enhancing public engagement and data collection.
Examples of AI in Scientific Research
To illustrate, here are some key applications, explained for a lay audience:
- Drug Discovery: AI simulates how different molecules interact with the body, helping identify potential drugs faster. For instance, it can predict which compounds might cure diseases like cancer, saving years of lab work (European Commission Artificial Intelligence (AI) in Science).
- Climate Science: AI models climate patterns using satellite data, predicting weather events and monitoring environmental changes. This helps in understanding climate change impacts, like ice melt or deforestation, aiding policy decisions.
- Genomics: AI analyzes millions of DNA sequences to find correlations with diseases, enabling personalized medicine. For example, it can identify genetic markers for conditions like Alzheimer’s, tailoring treatments to individuals.
- Astronomy: AI processes telescope data to discover new celestial bodies, expanding our understanding of the universe. It can identify galaxies or exoplanets in vast datasets, accelerating astronomical research.
- Materials Science: AI designs new materials with specific properties, like better batteries or superconductors, revolutionizing technology and energy storage.
These examples show AI’s transformative potential, making research deeper by uncovering insights that were previously inaccessible.
The Growing Interest and Controversy
The deep research trend is gaining traction, with projections like the AI agents market valued at USD 3.86 billion in 2023, expected to grow at 45.1% annually from 2024 to 2030, indicating significant investment in AI for research (DigitalOcean 7 Types of AI Agents to Automate Your Workflows in 2025). X posts from March 2025 also highlight AI’s role in scientific research, with mentions of its impact on healthcare and education, reflecting public and industry interest (X post).
However, there are controversies, particularly around ethics and regulation. Issues like data privacy, AI authorship in scientific papers, and the potential for bias in AI models are debated. For instance, some journals prohibit AI-generated images in manuscripts, while others require full disclosure of AI use, reflecting ongoing discussions (MIDAS User’s Guide – Generative AI Hub). These debates ensure responsible integration, balancing innovation with ethical considerations.
How to Begin Using AI in Research
For non-technical users, getting involved is easier than you might think. Citizen science projects that use AI allow anyone to contribute, democratizing research. Here are some platforms:
- iNaturalist: Upload photos of plants and animals to help train AI models for species identification (iNaturalist Citizen Science Platform).
- Zooniverse: Participate in projects like classifying galaxies or transcribing documents, aiding research efforts (Zooniverse Citizen Science Platform).
- Common Voice by Mozilla: Contribute voice data to train AI for speech recognition in various languages, enhancing global research (Nesta How AI might impact citizen science).
These projects are user-friendly, requiring no technical skills, and offer a way to see AI in action. For those curious to learn more, online courses or popular science books can provide insights, while businesses can explore AI tools for data analysis in marketing or operations.
Detailed Analysis and Future Outlook
To provide a structured overview, here’s a table summarizing key aspects of AI in scientific research:
Aspect | Description | Examples |
---|---|---|
Data Processing | AI analyzes vast datasets, identifying patterns humans might miss. | Genomic sequencing, climate modeling |
Prediction | AI predicts outcomes, speeding up research and reducing costs. | Drug efficacy, weather forecasts |
Experiment Design | AI designs experiments, optimizing research processes. | Material property testing |
Citizen Science Role | AI enables non-experts to contribute, enhancing data collection and engagement. | iNaturalist, Zooniverse |
Ethical Concerns | Debates around data privacy, AI authorship, and bias in models. | Journal policies on AI-generated content |
This table highlights AI’s multifaceted role, from technical capabilities to societal impact. The future outlook is promising, with AI expected to deepen research further, potentially leading to breakthroughs in fields like quantum computing or personalized medicine. However, ongoing ethical discussions will shape its responsible use, ensuring benefits are shared equitably.
Call to Action and Engagement
The deep research trend in AI is not just for scientists; it’s for everyone. By participating in citizen science, you can contribute to real-world research, seeing how AI transforms science. Stay informed through resources like Microsoft News Center or Fast Data Science, and explore how AI can enhance your own interests or work. The future of science is collaborative, and your involvement can make a difference.
Key Citations
- Microsoft News Center 6 AI trends you’ll see more of in 2025
- Fast Data Science AI in science and research
- European Commission Artificial Intelligence (AI) in Science
- Society for Science Research at Home: Citizen Science
- iNaturalist Citizen Science Platform
- Zooniverse Citizen Science Platform
- Nesta How AI might impact citizen science
- DigitalOcean 7 Types of AI Agents to Automate Your Workflows in 2025
- MIDAS User’s Guide – Generative AI Hub
- X post AI trends in 2025 include AI agents